Artificial Intelligence
20220812
Artificial Intelligence (AI) gets variously redefined over time. Alan Turing was a significant prophet for AI in the 1940βs. The origins as a field date from a workshop in 1956 at Dartmouth College in the USA funded by the Rockefeller Foundation. The workshop agreed on the term artificial intelligence and proposed that it encapsulate:
an attempt to find how to make machines use language, form abstractions and concepts, solve kinds of problems now reserved for humans, and improve themselves. β¦ For the present purpose the artificial intelligence problem is taken to be that of making a machine behave in ways that would be called intelligent if a human were so behaving.
That captures it nicely. Thereβs language for communications, representing knowledge as abstractions and concepts, solving problems that are the domain of humans, and learning. These are also at the core of human intelligence.
Data Science gained currency in 2014 to refer to the endeavour of using AI and Machine Learning (and Statistics) to gain new insights and knowledge from data. It aims to translate the fundamental research developments in AI and Machine Learning and making them available and applicable to real problems.
Since the 1950βs we have seen four seasons for AI. The 1950βs saw reasoning as search with developments in natural language, micro worlds, and neural networks. The 1980βs saw a surge in expert systems, knowledge representation, and back propagation. The 1990βs saw a focus on agents with Deep Blue, intelligent agents and the emergence of Data Mining. The 2010βs saw the emergence of massive data and massive compute, with data science and deep learning made possible by the coupling of accessible massive compute on the cloud and the centralised collection of massive amounts of personal data. The Future will see complex knowledge capture and reasoning, widespread access to AI, and massively distributed but privately managed data and personal agents analysing our data.
The current era continues to set the foundations for computing machines that will demonstrate AI, though that remains some way off. We see the foundations emerging from the research laboratories in universities and in industry. The MLHub shares the results of these developments, simply, within a framework that is easy for you to build models to share and a platform that makes it easy for anyone to explore and utilise the technology.
This bookβs scope is considerable, to cover the practical capabilities of all AI, machine learning and data science as it is today. Thatβs a pretty tall order and step-by-step we head towards that vision. In addition to the significant and useful tools we throw in a few whimsical tools for the fun of it.
Your donation will support ongoing availability and give you access to the PDF version of this book. Desktop Survival Guides include Data Science, GNU/Linux, and MLHub. Books available on Amazon include Data Mining with Rattle and Essentials of Data Science. Popular open source software includes rattle, wajig, and mlhub. Hosted by Togaware, a pioneer of free and open source software since 1984. Copyright © 1995-2022 Graham.Williams@togaware.com Creative Commons Attribution-ShareAlike 4.0
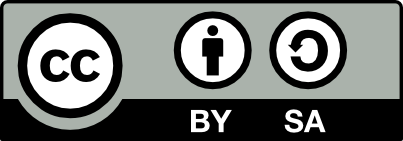