7.13 kmeans pipeline
20220220 As with all mlhub commands, a goal is to provide powerful combinations of commands through pipelines. We might process a csv file through a number of steps, for example to normalise the columns, to then pipe the csv file into the train command followed by the predict command to output a csv file with each observation labelled with a cluster number.
cat iris.csv | ml train kmeans 3 | ml predict kmeans iris.csv
The output will be something like:
sepal_length,sepal_width,petal_length,petal_width,label
5.0,3.6,1.4,0.2,1
7.7,3.8,6.7,2.2,0
6.1,3.0,4.9,1.8,2
5.4,3.7,1.5,0.2,2
...
The pipeline can go one step further to visualise the clustering:
cat iris.csv | ml train kmeans 3 | ml predict kmeans iris.csv | ml visualise kmeans
This will popup a window to display the clustering result.
TODO: Include the resulting plot here.
A pipeline including normalise can be
illustrated with the wine.csv
dataset from Section
7.14:
cat wine.csv |
ml normalise kmeans |
tee norm.csv |
ml train kmeans 4 |
ml predict kmeans norm.csv |
mlr --csv cut -f label |
paste -d"," wine.csv -
Here after normalising the input dataset the result is saved to a file
norm.csv
using tee whilst piping the same data on
to the next command (to train a clustering). We
save to file since we’d like to predict the
clusters for each of the normalised observations, then map them back
to the original observations. This is accomplished using a combination
of mlr to cut the label column from the csv
output from the predict command, and then we
paste that label column to the original wine.csv
.
The output is something like:
alcohol,malic,ash,alcalinity,magnesium,total,flavanoids,nonflavanoid,proanthocyanins,color,hue,...
14.23,1.71,2.43,15.6,127,2.8,3.06,.28,2.29,5.64,1.04,3.92,1065,0
13.2,1.78,2.14,11.2,100,2.65,2.76,.26,1.28,4.38,1.05,3.4,1050,0
...
13.4,3.91,2.48,23,102,1.8,.75,.43,1.41,7.3,.7,1.56,750,3
13.27,4.28,2.26,20,120,1.59,.69,.43,1.35,10.2,.59,1.56,835,3
13.17,2.59,2.37,20,120,1.65,.68,.53,1.46,9.3,.6,1.62,840,2
14.13,4.1,2.74,24.5,96,2.05,.76,.56,1.35,9.2,.61,1.6,560,2
Once again we can visualise the result as part of the pipeline, whilst also using tee to also save the clustering to file:
cat wine.csv |
ml normalise kmeans |
tee norm.csv |
ml train kmeans 4 |
ml predict kmeans norm.csv |
mlr --csv cut -f label |
paste -d"," wine.csv - |
tee clustering.csv |
ml visualise kmeans
TODO: Include the resulting plot here.
Your donation will support ongoing availability and give you access to the PDF version of this book. Desktop Survival Guides include Data Science, GNU/Linux, and MLHub. Books available on Amazon include Data Mining with Rattle and Essentials of Data Science. Popular open source software includes rattle, wajig, and mlhub. Hosted by Togaware, a pioneer of free and open source software since 1984. Copyright © 1995-2022 Graham.Williams@togaware.com Creative Commons Attribution-ShareAlike 4.0
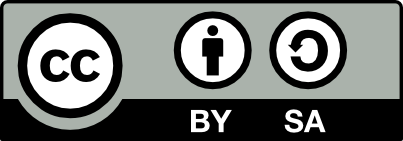